Generative artificial intelligence technologies are revolutionizing the day-to-day operations of enterprises. Unlike standard AI systems that rely on data from already-established systems, generative AI generates new data using methods such as natural language processing, machine learning, and deep learning. This cutting-edge technology is a game-changer for businesses, bringing automation to regular operations, simplifying processes, personalizing user experiences, and enhancing overall efficiency by delivering quick results. The benefits of using generative AI are many and businesses across a wide range of industries are quickly adopting it.
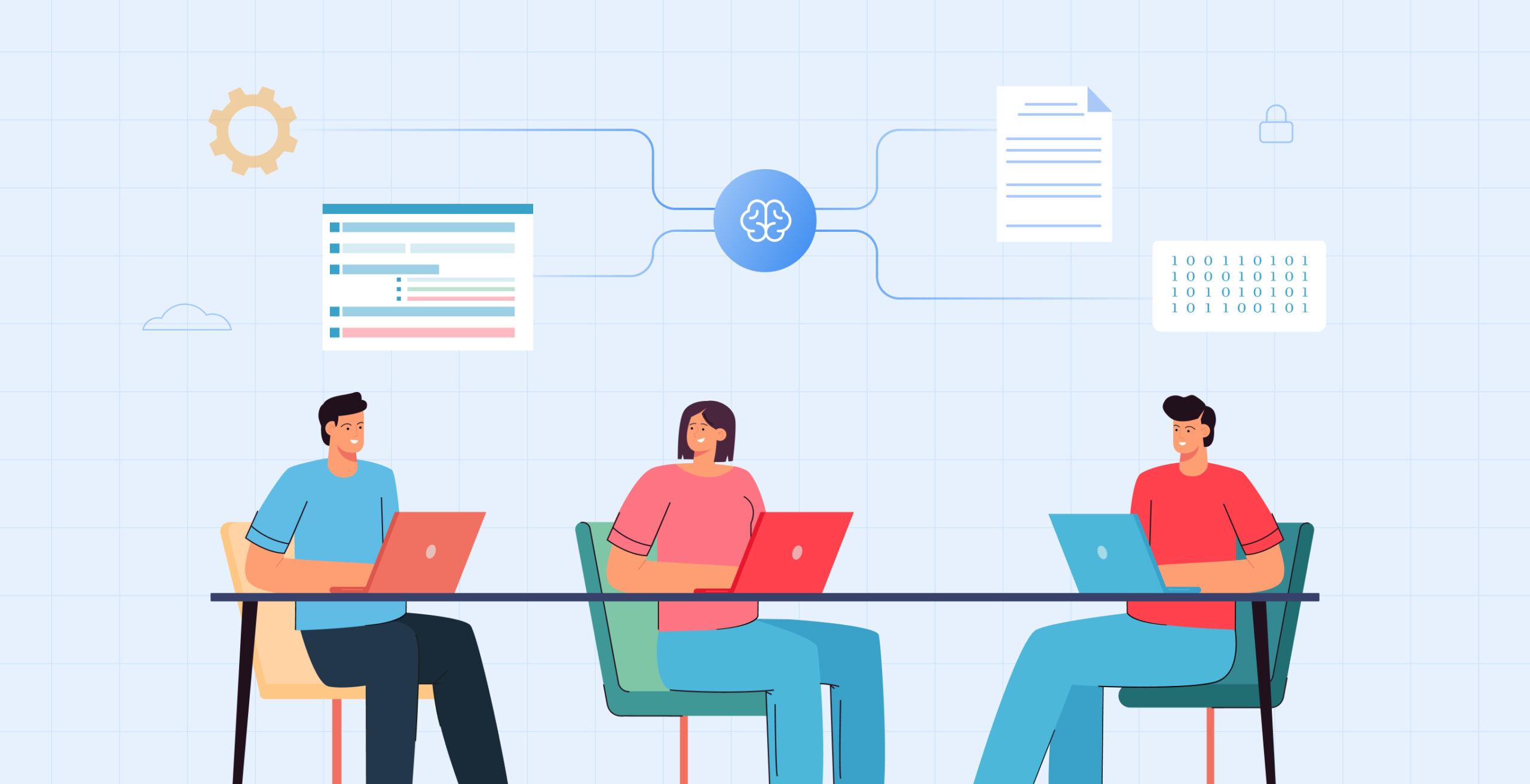
The influence of generative AI on the IT sector is enormous, and new applications are expected to emerge as the technology evolves. According to an article by VarIndia News, generative AI is rapidly evolving and set to revolutionize a number of industries, including IT. The global generative AI market is predicted to reach a staggering USD 111 billion by 2030, indicating the technology’s enormous potential.
One of the many advantages of generative AI is cost savings. Automating repetitive operations frees up IT workers’ time to focus on higher-level duties, enhancing efficiency and productivity. This, in turn, can help firms save both time and money in the long term. Another advantage of generative AI is its capacity to quickly and properly analyze massive volumes of data. Companies can become better educated to make data-driven decisions that can lead to higher revenue and cost savings.
In this blog, we will discuss the benefits and challenges of generative AI technology for IT teams. Additionally, we will explore how enterprise-ready generative AI technology is the ultimate solution to the current limitations of the technology.
What are the benefits of Generative AI for IT Teams
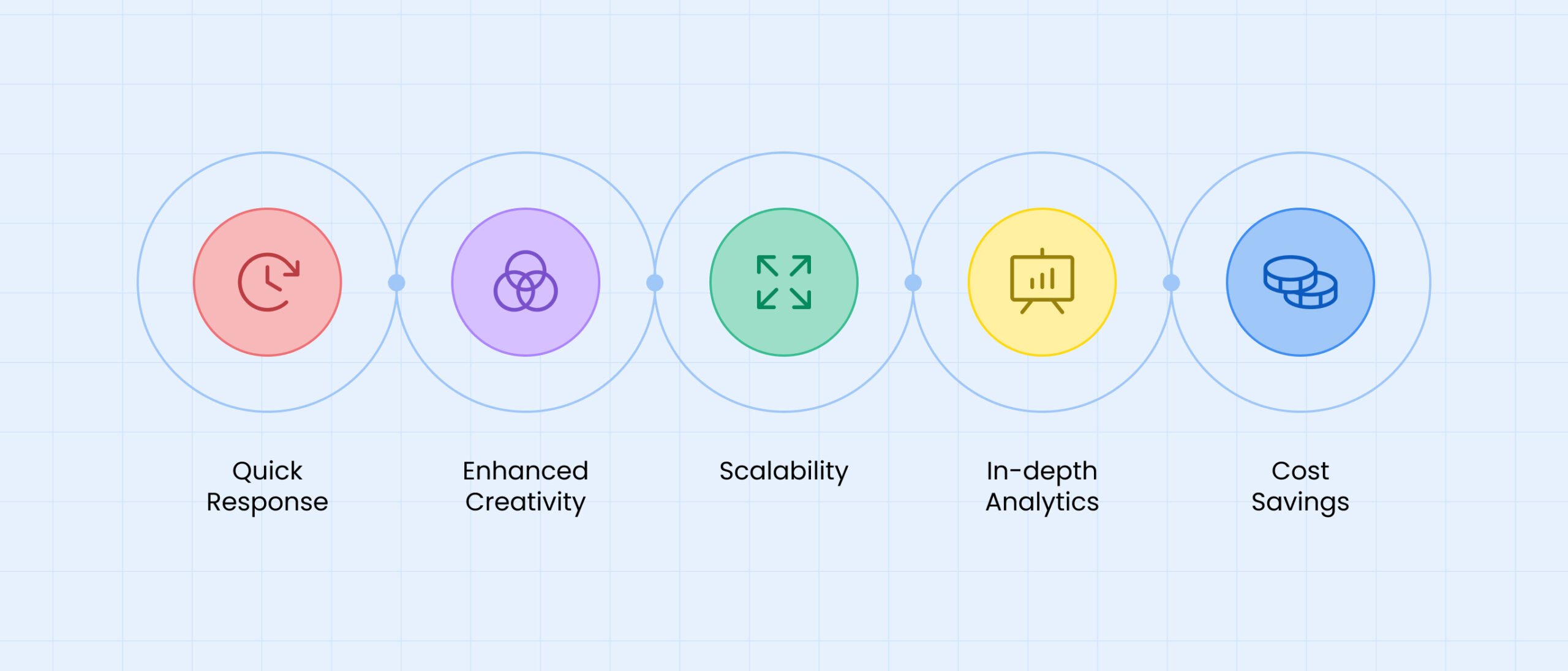
Generative AI technology has the potential to transform the IT sector by providing businesses with new tools and capabilities to create more efficient, creative, and personalized content. The technology, which can produce new material without the involvement of humans, provides various advantages in the field of information technology (IT), including the following:
Quick Response:
Standalone generative AI technology can produce content both more quickly and more efficiently than humans can, which may help organizations save both time and money in the process of content production.
Enhanced Creativity:
Generative AI technology can develop one-of-a-kind and inventive material that people may not have thought of, which may help organizations differentiate themselves from their rivals and produce content that is more engaging.
Scalability:
It is a feature of standalone generative AI technology that enables the creation of a high volume of material in a short amount of time. This enables organizations to better keep up with the needs of a digital world that is always shifting.
In-depth Analytics:
A Standalone Generative AI system can analyze enormous volumes of user data in order to develop personalized content that is suited to individual tastes and interests. This results in an improved level of personalization.
Cost Savings:
Because content may be generated by a standalone generative AI system without the need for input from humans, organizations have the potential to save money on labor costs and other expenses connected with conventional methods of content production.
Limitations of Generative AI for the IT Industry:
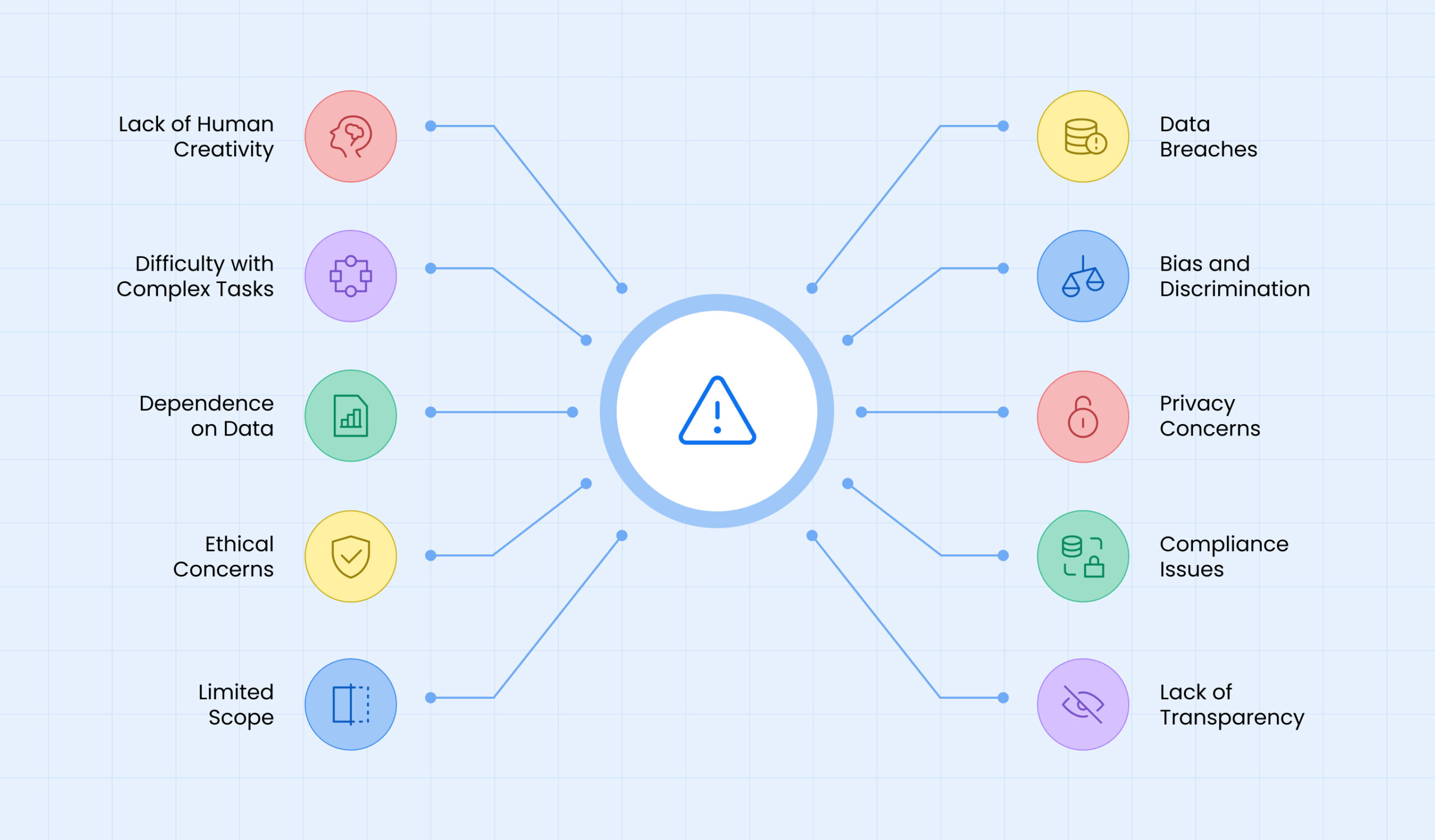
Lack of Human Creativity:
While generative AI technology can create unique content, it lacks the human touch and creativity that can make content truly stand out.
Difficulty with Complex Tasks:
Generative AI technology may struggle with complex tasks that require human-level understanding and decision-making skills, such as complex problem-solving or nuanced communication.
Dependence on Data:
Standalone generative AI technology relies heavily on data to generate content, which means that it may struggle in situations where data is incomplete, inaccurate, or biased.
Ethical Concerns:
The use of standalone generative AI technology raises ethical concerns around data privacy, ownership, and accountability, which must be addressed to ensure that businesses are using this technology responsibly.
Limited Scope:
Standalone generative AI technology is limited in scope and may not be suitable for all types of content creation tasks.
Data Breaches:
Storing large amounts of data required for generative AI algorithms can make businesses more susceptible to data breaches and cyber-attacks.
Bias and Discrimination:
Generative AI algorithms may learn biases and discriminatory practices from the data they are trained on, which can perpetuate harmful stereotypes and unfair practices.
Privacy Concerns:
Collecting and storing large amounts of user data for generative AI algorithms can raise privacy concerns, as users may not be comfortable sharing personal information with businesses.
Compliance Issues:
Businesses must comply with data privacy and security regulations such as GDPR, CCPA, and HIPAA, which can be challenging when using generative AI algorithms.
Lack of Transparency:
Generative AI algorithms are often considered to be “black boxes” because it can be difficult to understand how they generate their output, which can make it challenging to ensure compliance with data privacy and security regulations.
Addressing the limitations of Generative AI for IT teams:
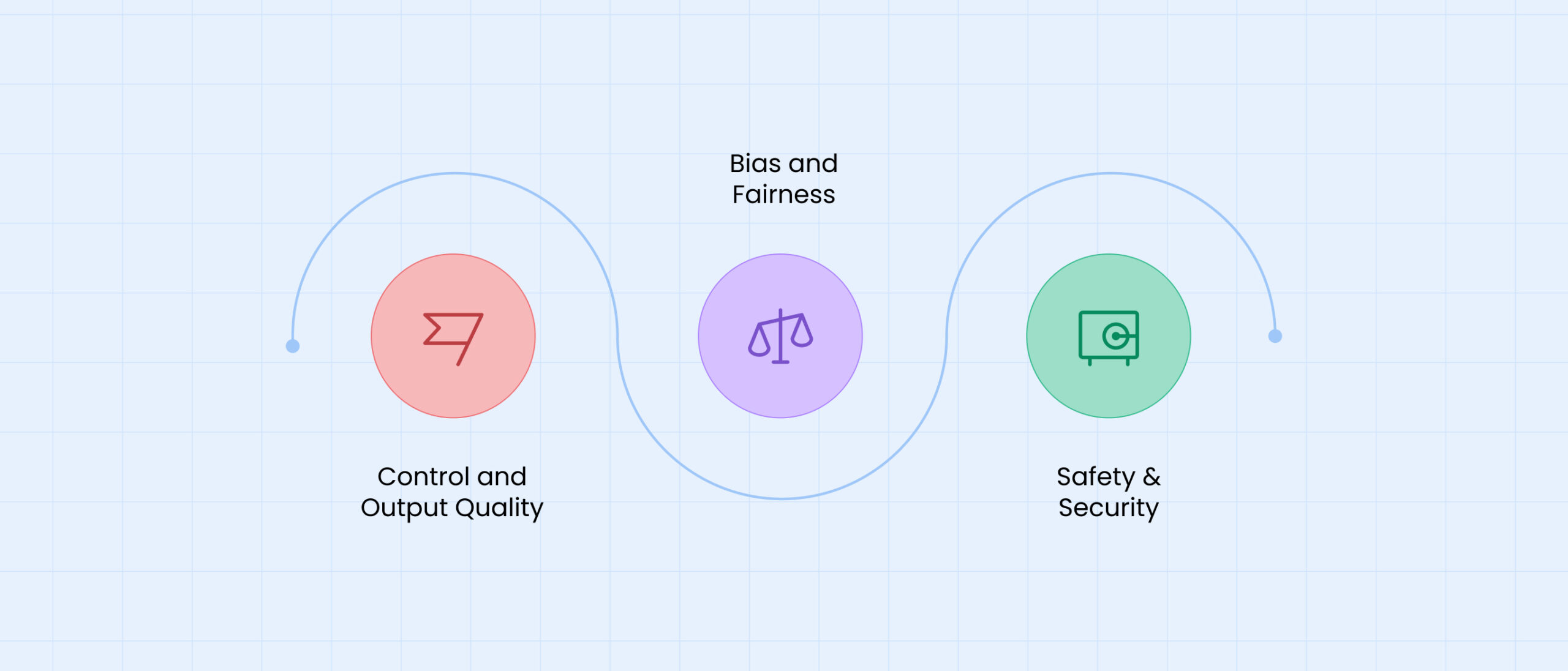
There are several approaches to overcoming the restrictions of employing independent generative AI technologies for IT teams. Because technology is still in its early stages, it is not suggested to rely entirely on it. So, let’s look at several approaches to overcoming the limits of generative AI technology:
Control and Output Quality:
To increase the quality of generated outputs, researchers can utilize more complex models, such as GANs and VAEs, which are better at capturing the underlying patterns and distributions of a dataset. Furthermore, training on bigger and more diverse datasets can aid in improving the quality of produced outputs. Researchers can utilize techniques like conditional GANs and VAEs to gain additional control over the features of generated outputs. These approaches allow the model to create outputs depending on a set of defined criteria.
Bias and Fairness:
To address bias and fairness, researchers can employ approaches like debiasing and fair representation learning, which can aid in the removal of biases contained in training data. Furthermore, researchers can employ approaches such as counterfactual data creation to provide more varied and representative training data.
Safety & Security:
Researchers may utilize strategies such as adversarial training and robust optimization to enhance the safety and security of generative AI systems. These approaches can assist to make the model more resistant to malicious assaults. Researchers can also employ techniques like watermarking and fingerprinting to establish provenance and trace the origins of created products.
Leena AI: The Enterprise-Ready Generative AI-powered tool for IT teams
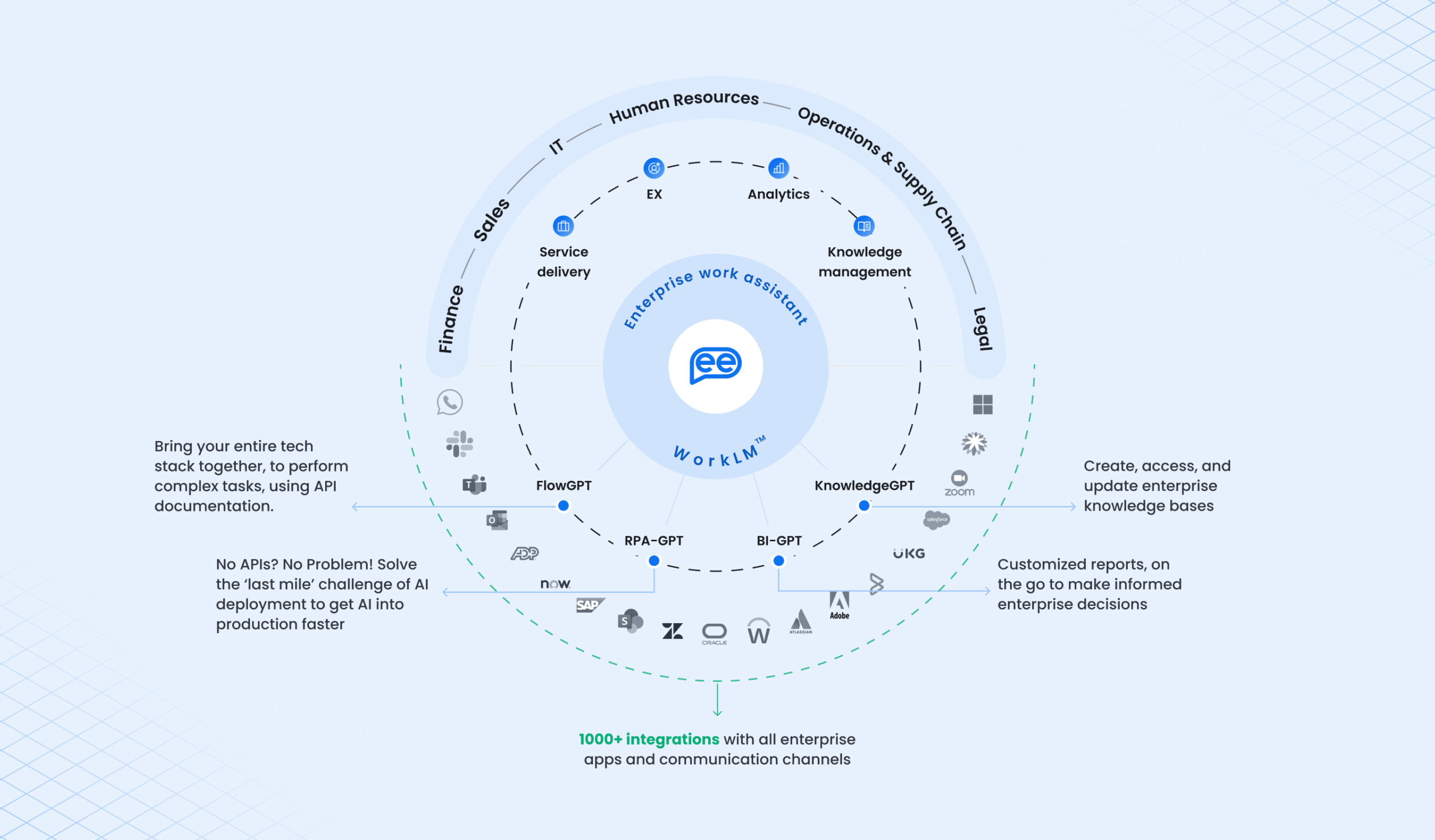
Introducing Leena AI’s WorkLMTM, a revolutionizing LLM for workplace automation powered by Generative AI technology. Our powerful LLM is built on GPT-J architecture, 7 billion parameters, and 2TB of proprietary data collected by Leena AI over 7 years. Leena AI’s WorkLMTM is a powerful tool that brings numerous benefits to IT teams. With its Generative AI capabilities, WorkLMTM efficiently resolves issues by quickly understanding and addressing complex problems, reducing downtime and improving productivity. It serves as an instant knowledge resource, providing step-by-step instructions, explanations, and relevant information to IT professionals, empowering them with instant access to valuable insights. Operating 24/7, WorkLMTM ensures scalability and availability, providing round-the-clock support even during non-business hours. Through continuous learning, WorkLMTM adapts to new technologies and industry trends, staying up to date and delivering increasingly accurate assistance. Moreover, WorkLMTM fosters collaboration within IT teams, serving as a central knowledge hub that facilitates information sharing and collaborative problem-solving. In summary, Leena AI’s WorkLMTM enhances IT team efficiency, productivity, and collaboration through its efficient issue resolution, instant knowledge access, scalability, continuous learning, and collaborative capabilities.
Conclusion
Generative AI has a lot of potential to revolutionize the IT sector, from changing the way businesses approach cybersecurity rules to automating monotonous work. Businesses that use this technology can gain a competitive edge by boosting efficiency and productivity, lowering expenses, and making more data-driven informed choices. Looking ahead, it seems obvious that generative AI will continue to improve, particularly in the domains of computer vision and natural language processing. This, in turn, will have a significant influence on the IT sector, and firms must be prepared to adapt to these changes in order to remain competitive.