Recruitment at organizations has evolved a lot and has become more data-driven in nature. From finding the right talent pool to shortlisting candidates and moving them along the recruitment funnel, data plays a critical role at every stage.
But when you look into HR processes, a lot of this data is being considered in the present. What about the information that can help in recruitment in the future?
That is why this blog highlights the importance of predictive analytics in recruitment.
What is predictive analytics in recruitment?
In simple terms, predictive analytics employs historical information, statistical data, and machine learning to make thoughtful and measured decisions to forecast future occurrences.
The objective is to provide the most accurate prediction of what will occur in the future and understand what has already happened.
For instance, imagine that an HR manager keeps track of the skills, location, travel, health, and behavior of a job seeker. With predictive analytics in recruitment, they can create a trajectory/ path of this applicant’s role in the organization and better predict if they are likely to be a value-add to the team. This data can also help managers determine how quickly the job seeker can exceed in their new role and the attrition in the upcoming months.
What can you measure and improve using predictive analytics in recruitment?
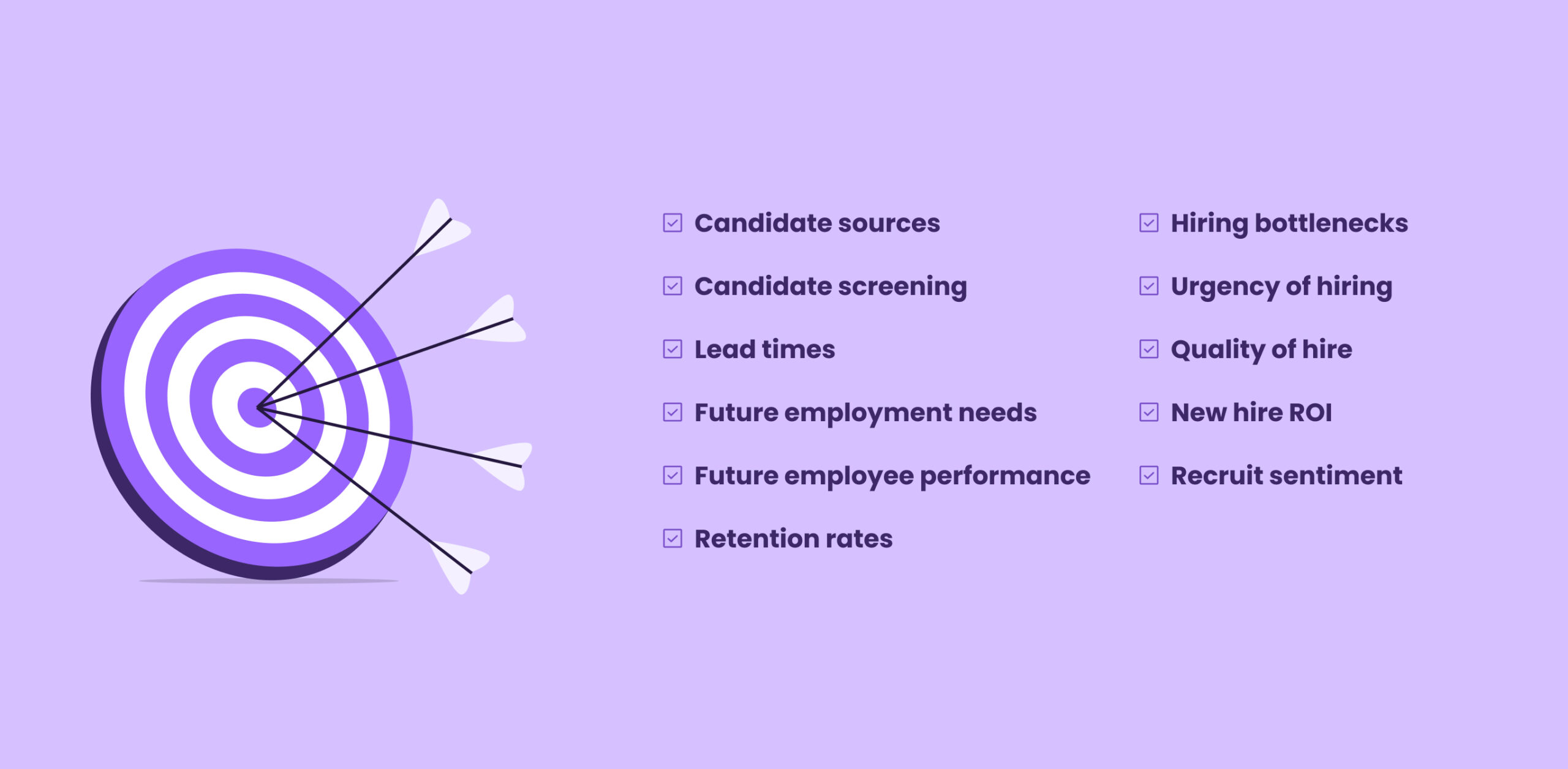
To make recruiting decisions based on data, all progressive firms use predictive analytics. It benefits both businesses and applicants by speeding up the hiring process and increasing the quality of hires.
Here are some of the things you can measure and improve with the help of predictive analytics in recruiting:
Candidate sources:
Predictive analytics can benchmark the channels you recruit through to highlight the most effective ones – referrals, job portals/boards, or other social media networking sites.
Candidate screening:
Predictive analytics aids in understanding how long the recruitment process takes and which candidate screening methods are the most efficient.
Lead times:
HR managers can measure the time between submitting an application, sending out an offer letter, and its acceptance, and how it affects drop-off rates to optimize efficiency.
Future employment needs:
Predictive analytics precisely evaluates what requirements the recruiting manager will have, the type of skills in demand, or the vacancy that will open soon.
Future employee performance:
Predictive analytics estimates the probability of a new applicant doing well in their role. It can also highlight the upskilling an employee may require to grow in their career.
Retention rates:
Predictive analytics also quantifies the length of time a recruit will work for the organization or the likelihood that additional applicants will reapply, helping businesses proactively tackle attrition.
Hiring bottlenecks:
When hiring process obstacles frequently arise, predictive analytics in recruitment can help understand them better. It can also help organizations identify ways and means to overcome and avoid them in the future.
The urgency of hiring:
Predictive analytics estimates the urgent positions and competencies required to ensure business continuity.
Quality of hire:
Predictive analytics can help process data around previous and new hires to compare varied parameters, including their skill set, ability to adapt to the new job role, tenure with the organization, and contribution towards the business goals. It can help benchmark the quality of hires, which helps improve future job postings.
New hire ROI:
Since predictive analytics can look into how a new hire progresses at work, benchmark it against previous data, and tally the same with business goals and objectives, it becomes easy to estimate new hire ROI. This helps businesses optimize their burn-on resources, streamlining them on those that add the most value.
Recruit sentiment:
Another untapped aspect that often causes churn in recruitment processes is the sentiment of applicants going through it. With predictive analytics, it also becomes possible to analyze the applicant’s engagement at every stage and understand positive/ negative sentiments that may impact their decisions. This helps create a more consciously crafted recruitment process.
Simply put, predictive analytics in recruitment is not just about making hiring more efficient and effective. It is also about enabling the organization and the employees to achieve the best outcome out of the process and the eventual hire.
Getting started with predictive analytics in recruitment
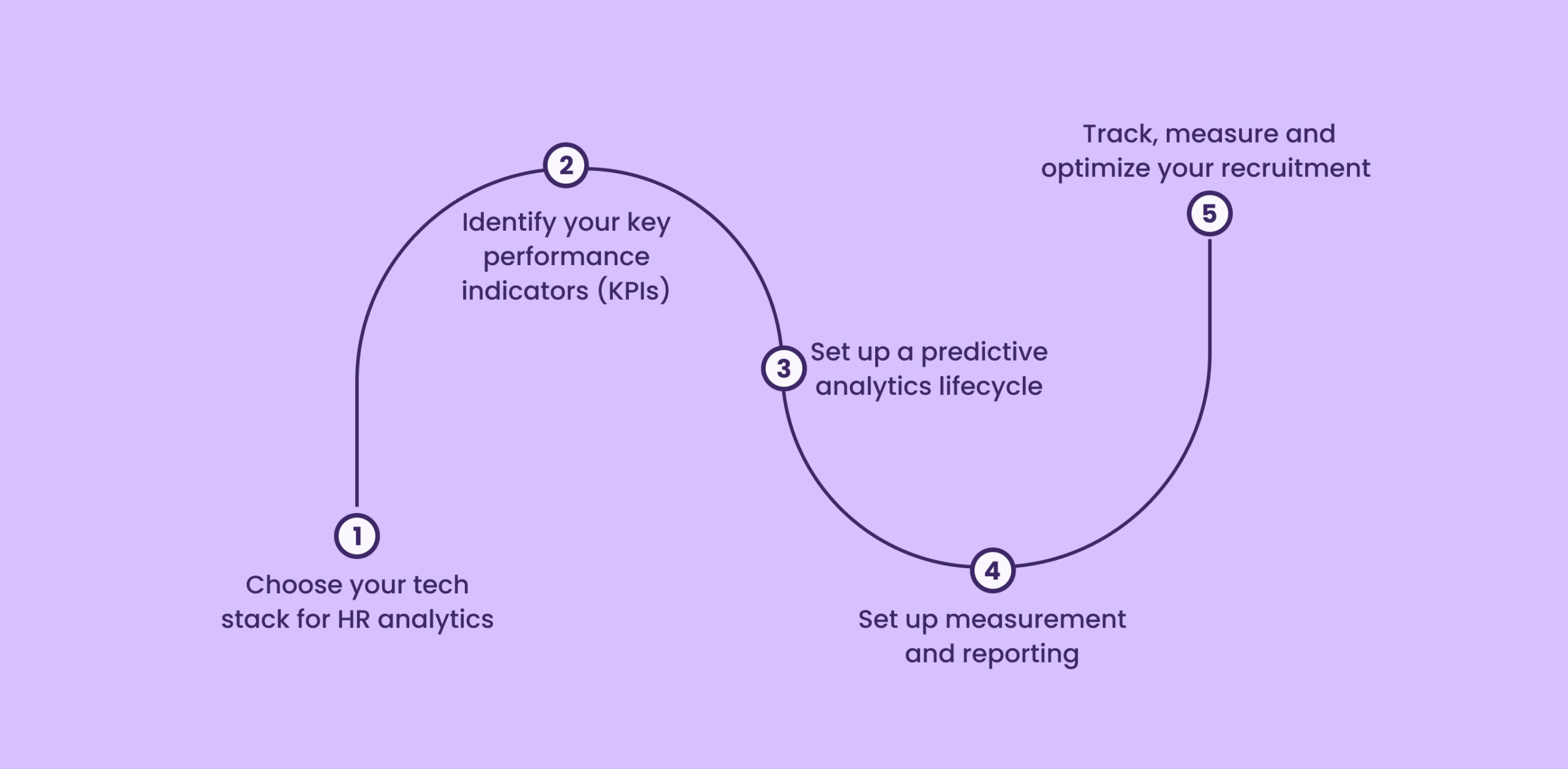
To leverage predictive analytics in recruitment, here are a few critical steps an organization is required to take.
Choose your tech stack for HR analytics
Every organization uses at least a handful of HR tools to streamline recruitment/ hiring, onboarding, and employee engagement. The very first step to making the most out of predictive analytics is to centralize all your data onto one dashboard. This step ensures that your ATS and HRIS are integrated with your IT stack.
While ATS is used to gather application data, HRIS (HR information system) is the software best suited to store, manage, and utilize employee data. This indicates that after a candidate gets hired, the function of the ATS system ceases, and the HRIS software takes over. With predictive analytics in place, the data that flows between the two stages through these systems – pre, and post-hiring, becomes more intelligent.
For instance, Leena AI is a comprehensive employee experience platform that uses artificial intelligence and machine learning to offer predictive analytics on all processes starting from recruitment.
Identify your key performance indicators (KPIs)
Predictive analytics unlocks a plethora of data for organizations. To make the most of the available insights and information, you should start with clearly defined business goals and objectives – this may vary based on business to business and the process you want to optimize.
For example, are you looking at improving your candidate sources, improving your referrals, optimizing your job profiles, planning your hires, or improving your retention rate of new hires? The more specific you are, the better you can make the most of predictive analytics.
Set up a predictive analytics lifecycle
Once you have your HR tech stack in place and your goals clearly laid out, the next step is to set up a predictive analytics lifecycle. A typical cycle looks as follows:
- Gathering data
- Data pre-processing (cleaning)
- Choosing a type of analysis
- Improving the model
- Executing forecasts
- Implementing crucial insights
- Measure and optimize
A good HR predictive analytics tool does most of the heavy lifting for you here. You need to clearly define the data source and health and then focus on implementing the changes recommended by predictive analytics.
Another vital aspect is that setting up a lifecycle may require a team effort, which may spread across multiple departments. It is crucial to clearly define who is a stakeholder on the basis of the goals and objectives set.
Set up measurement and reporting
The next step is to develop a dashboard with recruiting KPIs to track the outcomes. Ensure that the dashboard is user-friendly and straightforward to use. Furthermore, it should contain only the necessary data for your primary KPIs. Your tasks will be more precise in the long run if your HR stack can deliver speedy and transparent reports.
You may choose what information you want to view and how it gets displayed using platforms like Leena AI, which comes with an analytics dashboard with advanced visualization. This makes it easier not just to map data but also to understand its implications.
It is also a good idea to set a clear cadence for reporting – weekly, biweekly, monthly, and yearly. This is important to get everyone on the same page and be able to benchmark improvements with the use of predictive analytics.
Track, measure, and optimize your recruitment
Predictive analytics provide future-focused information. Due to its emphasis on probability and effect, it can be tailored to meet the company’s demands, goals, and objectives.
But to do so consistently, it is essential to create a team that is held accountable for the KPIs set for the same. This way, you can ensure that you are not just using predictive analytics to uncover insights but proactively optimizing the recruitment process also for better outcomes.
Making the most of predictive analytics in recruitment
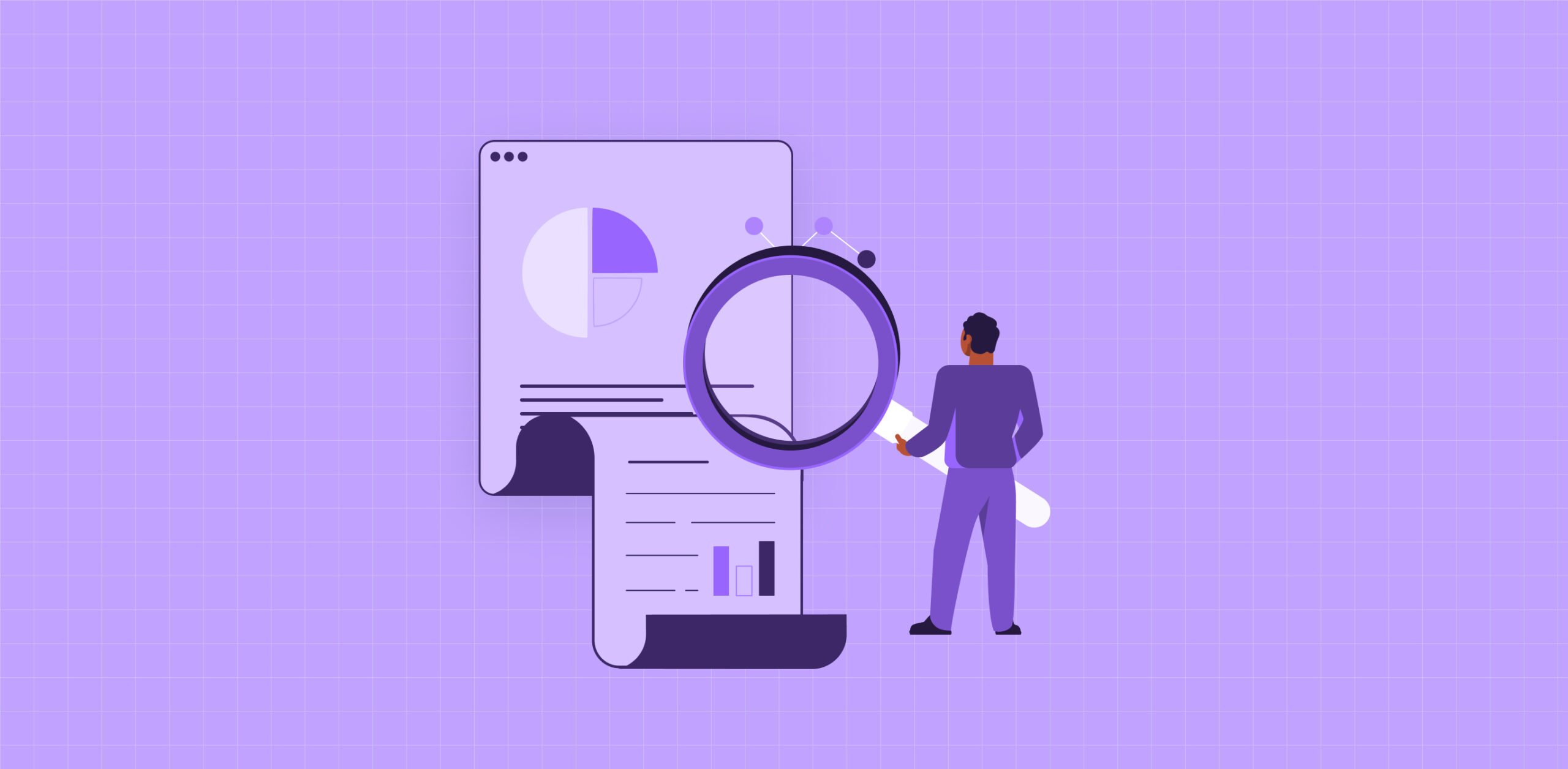
By seeing trends and patterns in your data, predictive analytics can detect possible risk areas in your recruitment process and estimate how they can affect your hiring and the overall organizational goals.
For example, with the help of analytics, organizations can prepare for unforeseen events like the pandemic that eventually led to a shift in work cultures and an entire period of great resignation. It can help them understand the changing needs and preferences of the talent pool, optimizing not just the offer letters but how they engage them from day one, leading to favorable outcomes on both ends.
Ready to get started with using predictive analytics in recruitment? Book a demo of Leena AI today.